Unraveling the Correlation between Raman and Photoluminescence in Monolayer MoS2 through Machine Learning Models

Dr. Ang-Yu Lu
PhD Student
Department of Electrical Engineering and Computer Science, Massachusetts Institute of Technology
*The organization and the title are those when awarded
Research summary
Two-dimensional (2D) transition metal dichalcogenides (TMDCs)*1 with intense and tunable photoluminescence (PL) *2 have opened up new opportunities for optoelectronic and photonic applications such as light-emitting diodes, photodetectors, and single-photon emitters. Among the standard characterization tools for 2D materials, Raman spectroscopy stands out as a fast and non-destructive technique capable of probing material crystallinities and perturbations, such as doping and strain. However, due to its highly nonlinear nature, a comprehensive understanding of the correlation between PL and Raman spectra in monolayer MoS2 remains elusive.
In this work, Dr. Lu has systematically explored the connections between PL signatures and Raman* modes, providing comprehensive insights into the physical mechanisms correlating PL and Raman features. This analysis further disentangles the strain and doping contributions from the Raman spectra through machine-learning models. First, he deployed a DenseNet*to predict PL maps using spatial Raman maps*. Moreover, he applyed a gradient-boosted trees model (XGBoost)* with Shapley additive explanation (SHAP)* to evaluate the impact of individual Raman features on PL features, allowing him to link the strain and doping of monolayer MoS2. Lastly, Dr. Lu adopted a support vector machine (SVM)* to project PL features onto Raman frequencies. This work may serve as a methodology for applying machine learning in 2D material characterizations and providing the knowledge for tuning and synthesizing 2D semiconductors for high-yield PL.
Compounds consisting of transition metal elements and chalcogen elements such as sulfur, selenium, and tellurium, which have a two-dimensional layered structure.
A phenomenon in which electrons in a material absorb light and emit light specific to that material.
A phenomenon in which the crystal lattice of a material scatters light specific to that material. The scattered light can be spectrally analyzed to determine the characteristics of the material.
A convolutional neural network used to learn features of images and patterns. Convolution refers to the process of detecting local patterns within an image.
A mapping of Raman spectra obtained from different positions on the surface of a material using Raman spectroscopy.
A machine learning algorithm known as gradient boosting.
A method for evaluating the contribution of features to the predictions of a machine learning model.
A machine learning algorithm used for tasks such as classification and regression.
Compounds consisting of transition metal elements and chalcogen elements such as sulfur, selenium, and tellurium, which have a two-dimensional layered structure.
A phenomenon in which electrons in a material absorb light and emit light specific to that material.
A phenomenon in which the crystal lattice of a material scatters light specific to that material. The scattered light can be spectrally analyzed to determine the characteristics of the material.
A convolutional neural network used to learn features of images and patterns. Convolution refers to the process of detecting local patterns within an image.
A mapping of Raman spectra obtained from different positions on the surface of a material using Raman spectroscopy.
A machine learning algorithm known as gradient boosting.
A method for evaluating the contribution of features to the predictions of a machine learning model.
A machine learning algorithm used for tasks such as classification and regression.
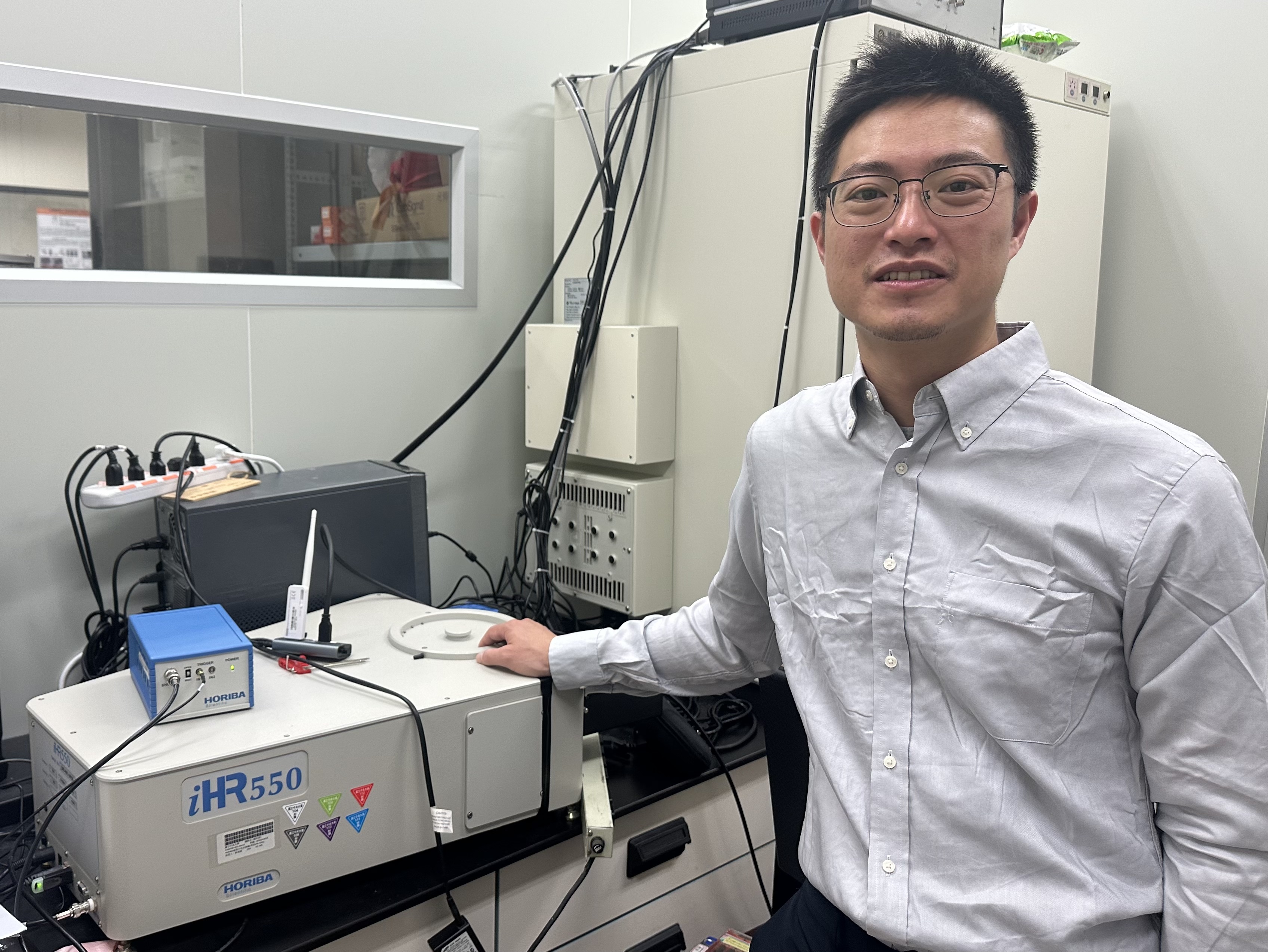
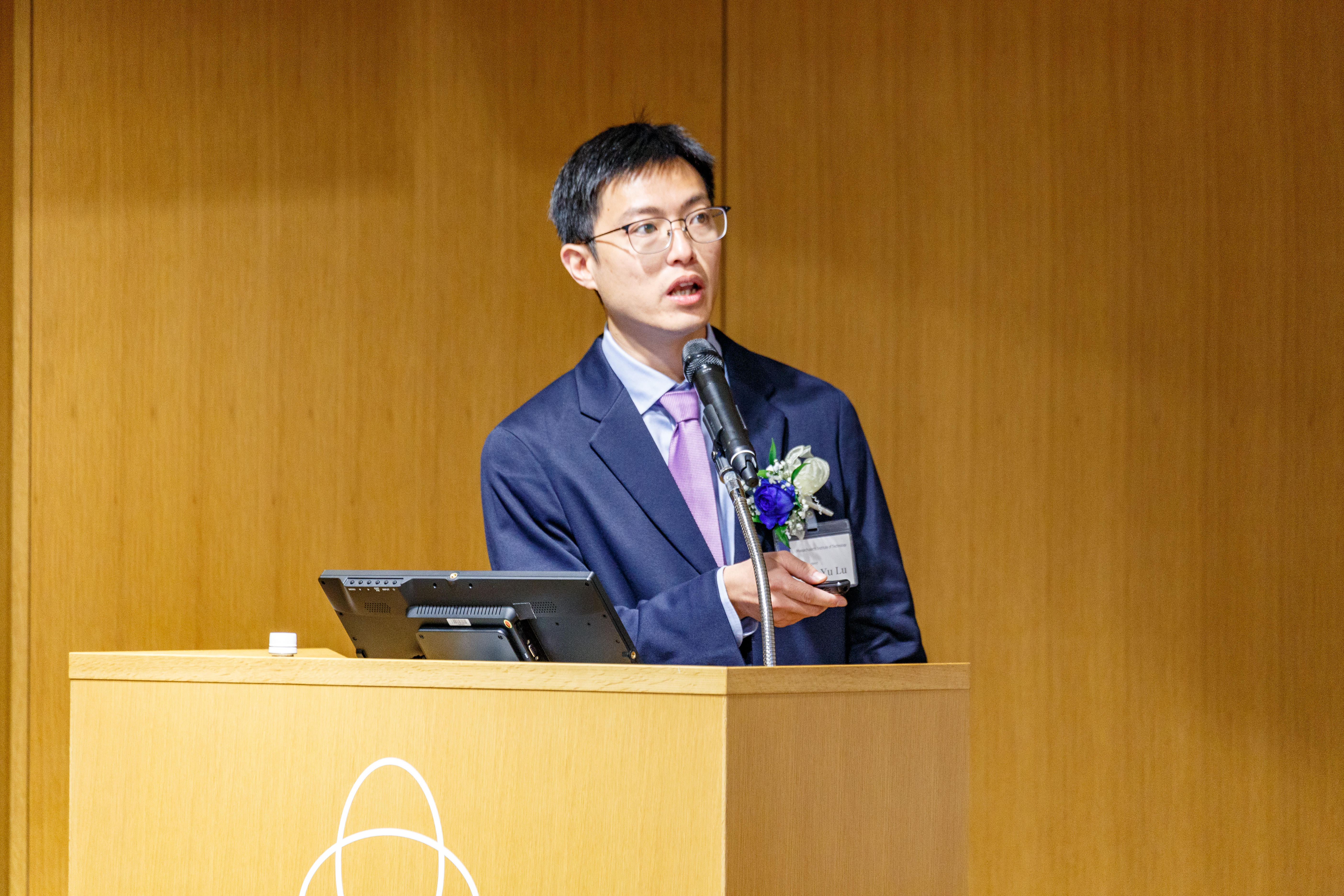